Navigating unknown environments to find a target object is a significant challenge. While semantic information is crucial for navigation, relying solely on it for decision-making may not always be efficient, especially in environments with weak semantic cues. Additionally, many methods depend on the single-frame output of an object detector for target identification, making them vulnerable to misdetections, particularly in scenes with visually similar objects. To address these limitations, we propose ApexNav, a zero-shot object navigation framework that is both more efficient and reliable. For efficiency, we introduce an adaptive exploration strategy that alternates between semantic-based and geometry-based exploration. It prioritizes semantic exploration when strong cues are available and shifts to geometry-based exploration when semantic cues are weak, using geometric information to quickly gather environmental data. For reliability, we propose a target-centric semantic fusion method that preserves long-term memory of the target object and similar objects, reducing false detections and minimizing task failures. We evaluate ApexNav on the HM3D-v0.1, HM3D-v0.2, and MP3D datasets, where it outperforms state-of-the-art methods in both SR and SPL metrics. Comprehensive ablation studies further demonstrate the effectiveness of each module. [Video]
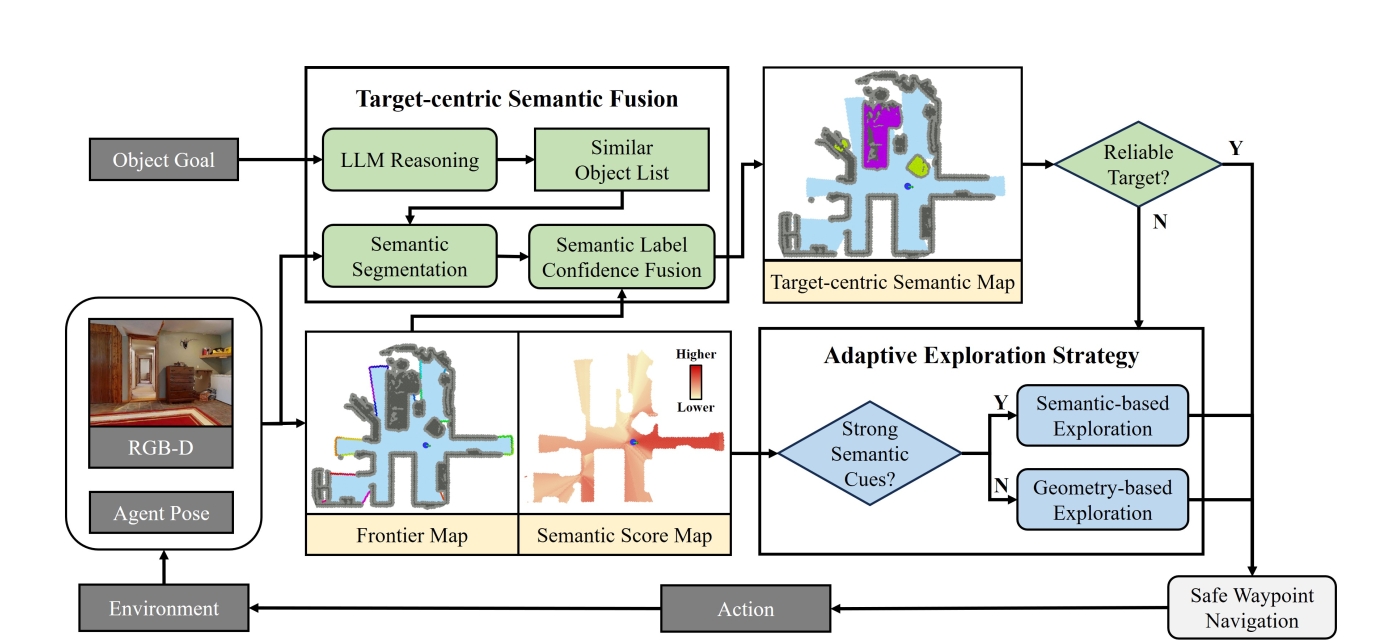
Communication is fundamental for multi-robot col-laboration, with accurate radio mapping playing a crucial role in predicting signal strength between robots. However, modeling radio signal propagation in large and occluded environments is challenging due to complex interactions between signals and obstacles. Existing methods face two key limitations: they struggle to predict signal strength for transmitter-receiver pairs not present in the training set, while also requiring extensive manual data collection for modeling, making them impractical for large, obstacle-rich scenarios. To overcome these limitations, we propose FERMI, a flexible radio mapping framework. FERMI combines physics-based modeling of direct signal paths with a neural network to capture environmental interactions with radio signals. This hybrid model learns radio signal propa- gation more efficiently, requiring only sparse training data. Additionally, FERMI introduces a scalable planning method for autonomous data collection using a multi-robot team. By increasing parallelism in data collection and minimizing robot travel costs between regions, overall data collection efficiency is significantly improved.
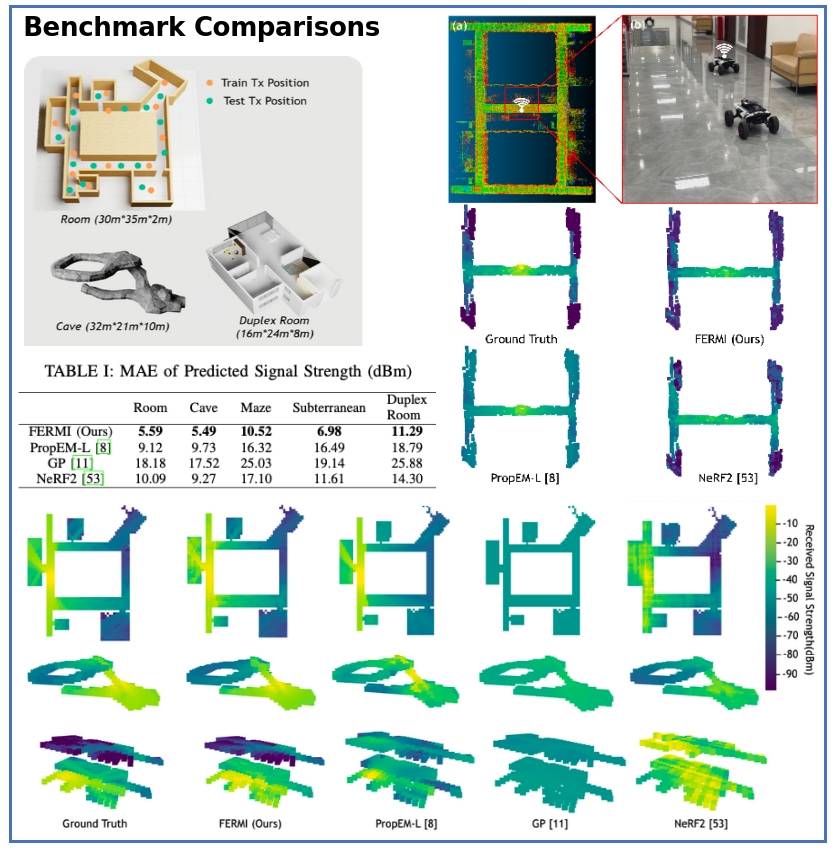
We propose DynamicPose, a real-time and robust 6D object pose tracking framework that handles fast-moving camera and object without retraining. To ensure accurate translation initialization, we introduce an efficient translation compensation mechanism that corrects Region of Interest shifts caused by rapid camera or object motion. Additionally, we design a VIO-guided Kalman filter with dynamically scaled multi-candidate refinement, enabling robust 6D pose tracking even under extreme rotations. Extensive experiments show that DynamicPose outperforms existing state-of-the-art(SOTA) methods for 6D object pose tracking in fast-moving camera and object scenarios, where the relative motion between the target object and the camera exceeds 1.5m/s and 3.0rad/s. [Video]
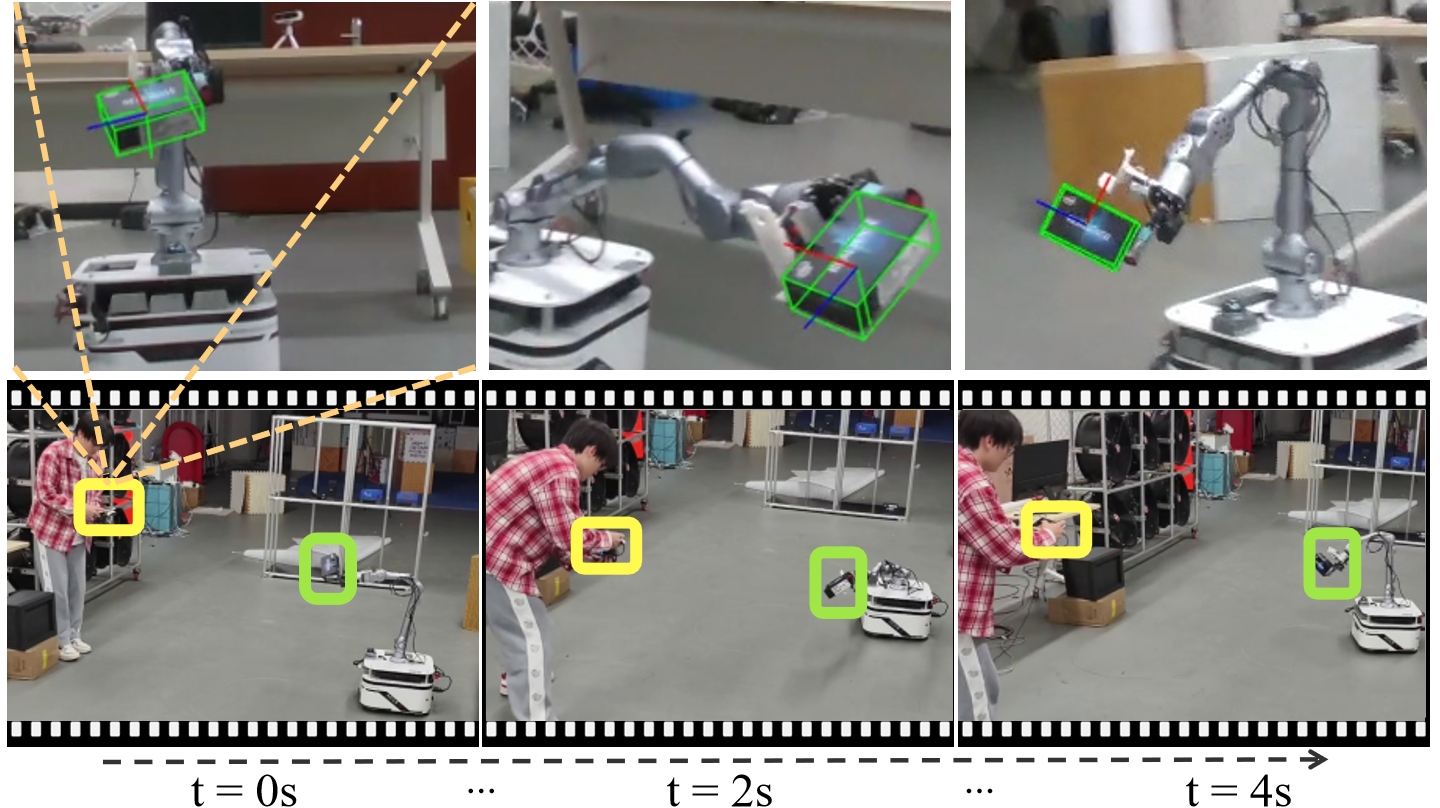
We propose a perception-aware planning method for quadrotor flight in unknown and feature-limited environments. Existing methods lack a systematic mechanism to allocate perception resources and efficiently integrate incrementally discovered features and unknown regions into planning, leading to collisions and high computation. We introduce a viewpoint transition graph to adaptively select local target viewpoints, guiding the UAV toward the goal while maintaining sufficient localizability and avoiding feature-limited regions. For trajectory generation, we construct localizable corridors via feature co-visibility evaluation as concise constraints, enabling efficient optimization that increases unknown information gain while preserving localization. Our method achieves faster and safer navigation with efficient replanning in unknown and feature-limited environments. [Video]
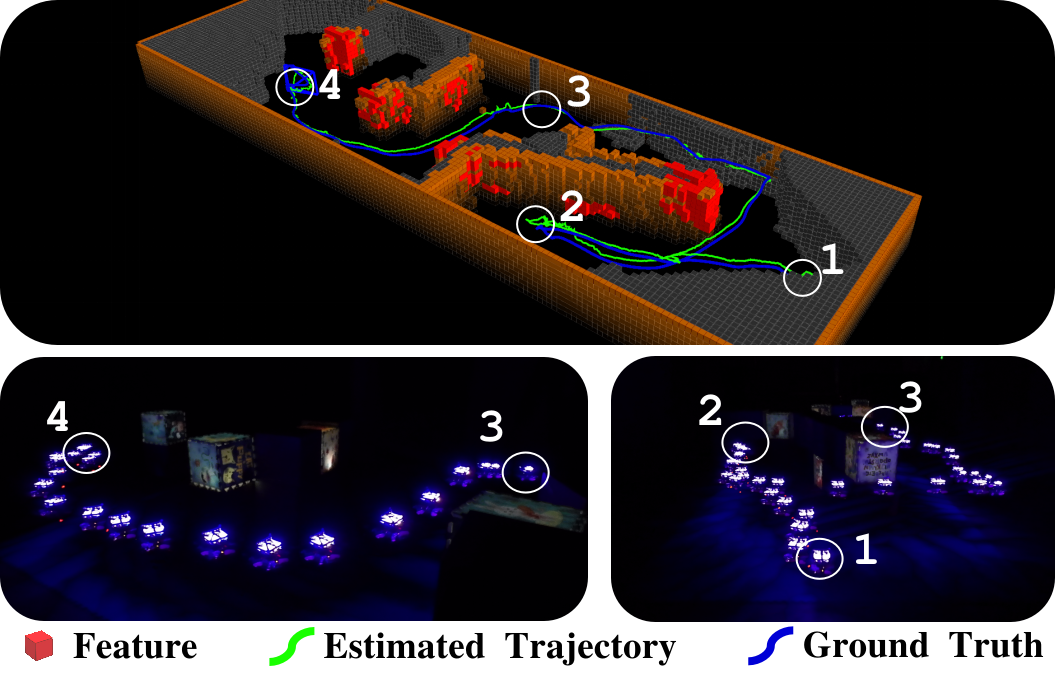
This paper presents EPIC, a lightweight LiDAR-based framework addressing challenges in UAV autonomous exploration. Traditional methods often require memory-heavy occupancy grids for frontier detection and struggle with computationally expensive path planning directly on point clouds. EPIC overcomes this by introducing a novel observation map based on point cloud quality, tracking well-observed versus poorly-observed areas using spatial hashing, thus eliminating global grids. It also features an incremental topological graph built directly on point clouds for efficient, real-time path planning. Combined in a hierarchical structure, these components enable agile, energy-efficient trajectories, achieving faster exploration with significantly reduced memory and computation compared to state-of-the-art methods in diverse environments. (Estimate: ~120 words, likely around 200 characters). [Video:Bilibili]
This paper tackles the challenge of autonomous target search using unmanned aerial vehicles (UAVs) in complex unknown environments. To fill the gap in systematic approaches for this task, we introduce Star-Searcher, an aerial system featuring specialized sensor suites, mapping, and planning modules to optimize searching. Path planning challenges due to increased inspection requirements are addressed through a hierarchical planner with a visibility-based viewpoint clustering method. This simplifies planning by breaking it into global and local sub-problems, ensuring efficient global and local path coverage in real time. Furthermore, our global path planning employs a history-aware mechanism to reduce motion inconsistency from frequent map changes, significantly enhancing search efficiency. [Video:Bilibili]
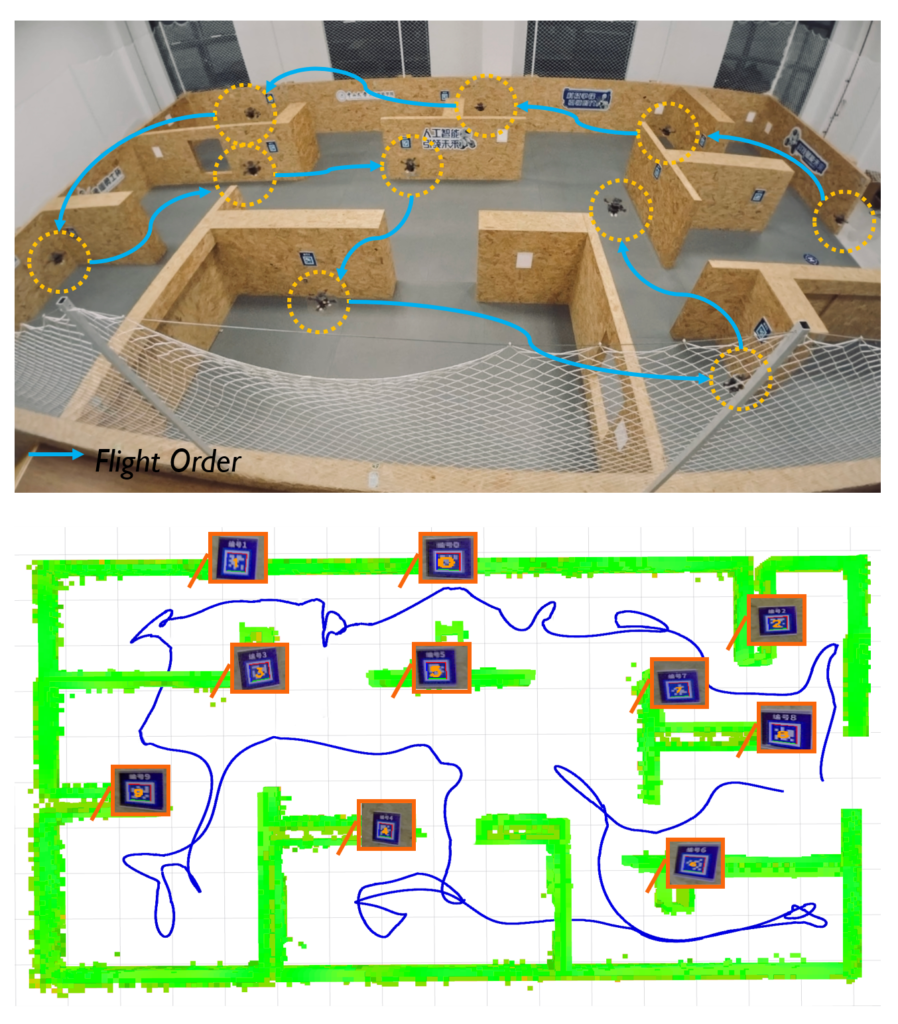
Recently, we present APACE, an Agileand Perception-Aware trajeCtory gEneration framework for quadrotors aggressive flight, that takes into account feature matchability during trajectory planning. We seek to generatea perception-aware trajectory that reduces the error of visual-based estimator while satisfying the constraints on smoothness, safety, agility and the quadrotor dynamics. The perception objective is achieved by maximizing the number of covisible features while ensuring small enough parallax angles. Additionally, we propose a differentiable and accurate visibility model that allows decomposition of the trajectory planning problem for efficient optimization resolution (ICRA 2024 Submission). [Video:Bilibili]
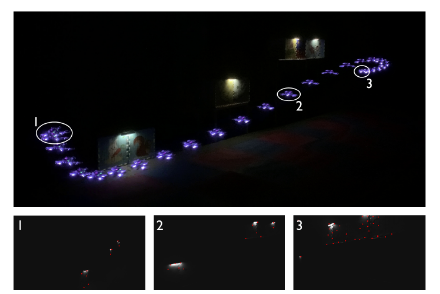
Mobile manipulators have recently gained significant attention in the robotics community due to their superior potential in industrial and service applications. However, the high degree of freedom associated with mobile manipulatorsposes challenges in achieving realtime whole body motion planning. To bridge the gap, this paper presents a motion planning method capable of generating high-quality, safe, agileand feasible trajectories for mobile manipulators in real time (ICRA 2024 Submission). [Video:Bilibili]
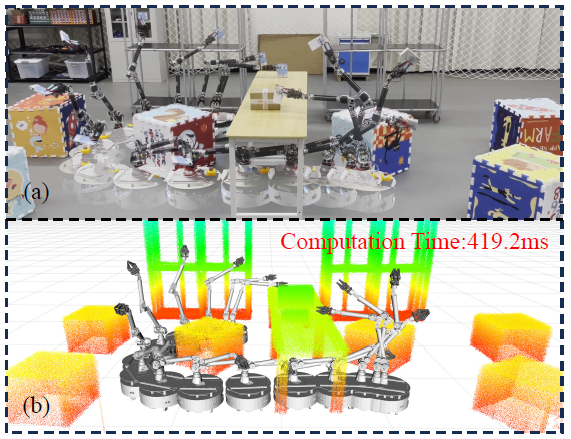
Recently, we propose FC-Planner, a skeleton-guided planning framework that can achieve fast aerial coverage of complex 3D scenes without pre-processing. We decompose the scene into several simple subspaces by a skeleton-based space decomposition (SSD). Additionally, the skeleton guides us to effortlessly determine free space. We utilize the skeleton to efficiently generate a minimal set of specialized and informative viewpoints for complete coverage. Based on SSD, a hierarchical planner effectively divides the large planning problem into independent sub-problems, enabling parallel planning for each subspace. The carefully designed global and local planning strategies are then incorporated to guarantee both high quality and efficiency in path generation. We conduct extensive benchmark and realworld tests, where FC-Planner computes over 10 times faster compared to state-of-the-art methods with shorter path and more complete coverage (ICRA 2024 Submission). [Video:Bilibili]
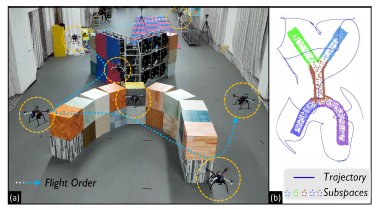
Recently, we propose MASSTAR: a multi-modal large-scale scene dataset with a versatile toolchain for surface prediction and completion. We collect a large amount of scene-level models including part of real-world captured data from a wide range of open-source works. A toolchain is also developed to facilitate processing the data by segmenting the raw 3D data and selecting the valuable model from raw 3D data and generating multi-modal data including RGB image, descriptive text, depth image, and partial point cloud. Additionally, we benchmark different algorithms trained on our dataset (ICRA 2024 Submission). [Video:Bilibili]
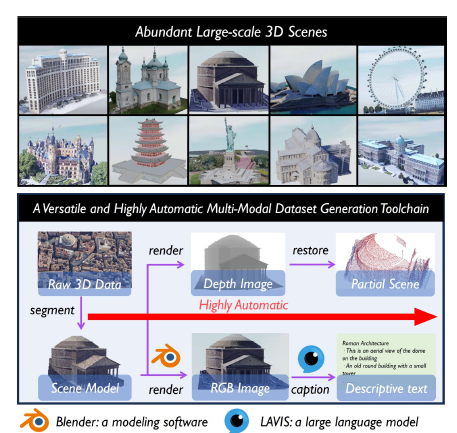
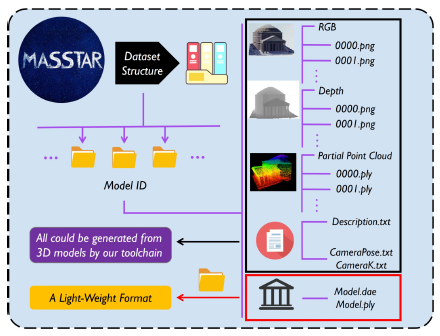
Recently, we propose a NeRF-based mapping method that enables higher-quality reconstruction and real-time capability even on edge computers of handheld devices and quadrotors by Chenxing JIANG and Hanwen ZHANG. Specifically, we propose a novel hierarchical hybrid representation and a coverage-maximizing keyframe selection strategy. Extensive experiments show our method achieves superior mapping results with less runtime compared to existing NeRF-based mapping methods. To the best of our knowledge, our method is the first to run a NeRF-based mapping method onboard in real-time.[Paper][Video:Bilibili][Video:Youtube][Code]
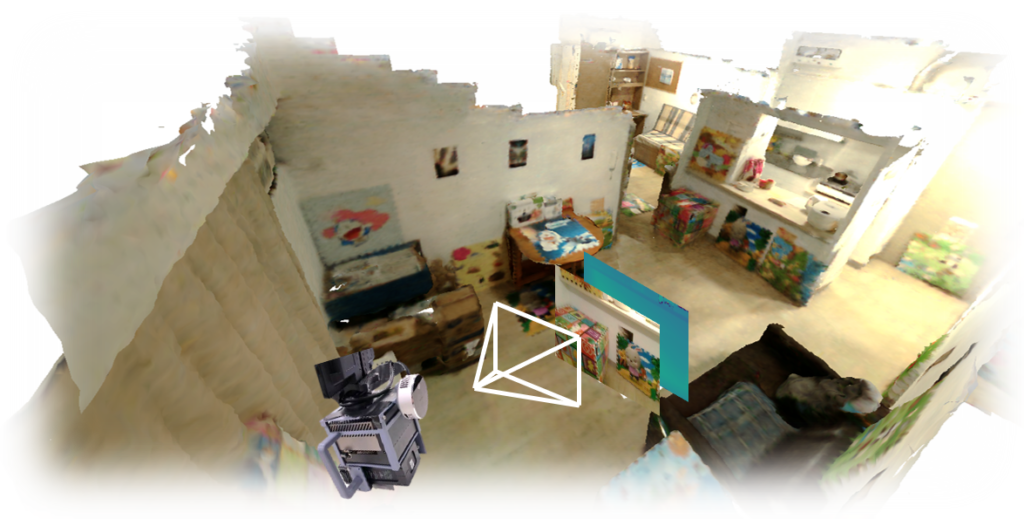
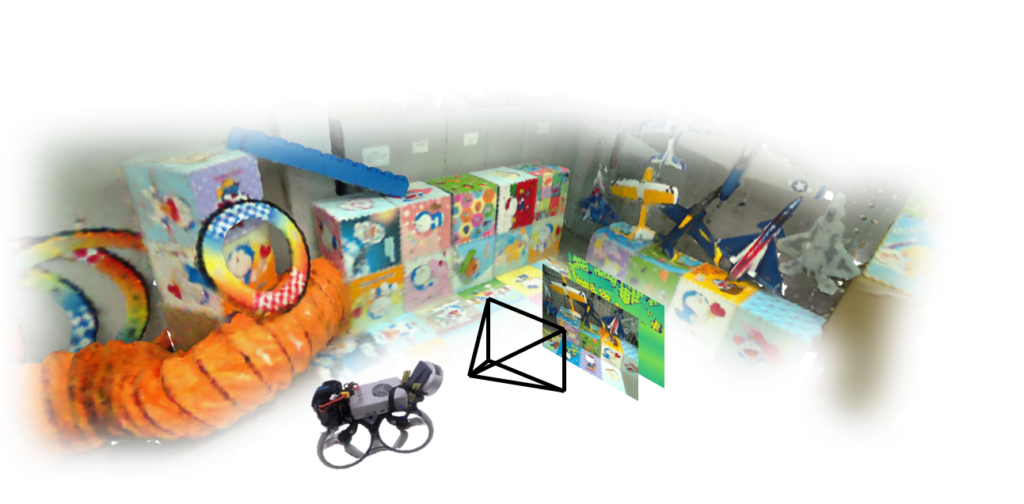
Recently, we developed a real-time planning method for UAV payload system considering the time-varying shape and non-linear dynamics to ensure whole-body safety and dynamic feasibility by Haojia Li. Additionally, an adaptive NMPC with a hierarchical disturbance compensation strategy is designed to overcome unknown external perturbations and inaccurate model parameters. Extensive experiments show that our method is capable of generating high-quality trajectories online, even in highly constrained environments, and tracking aggressive flight trajectories accurately, even under significant uncertainty. [Video]
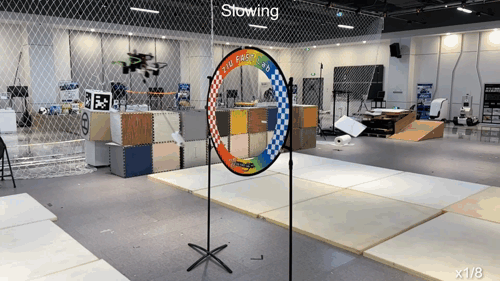
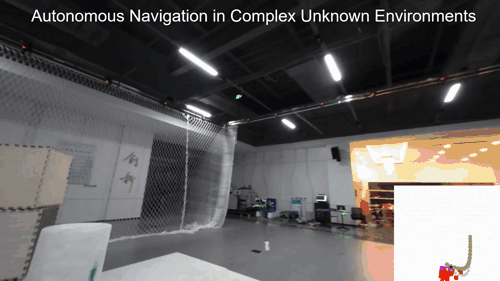
Recently, we further develop a fully decentralized approach for exploration tasks using a fleet of quadrotors. The quadrotor team operates with asynchronous and limited communication, and does not require any central control. The coverage paths and workload allocations of the team are optimized and balanced in order to fully realize the system’s potential. The associated paper has been published at IEEE T-RO. [Paper][Video][Code]
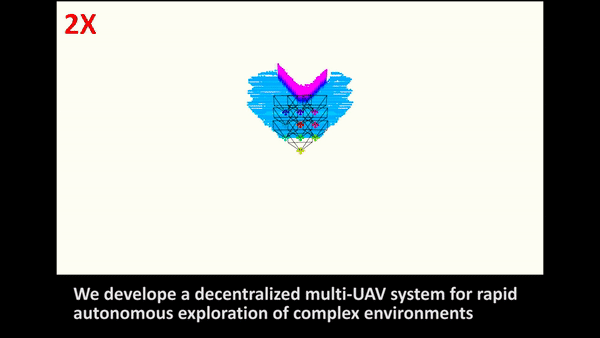
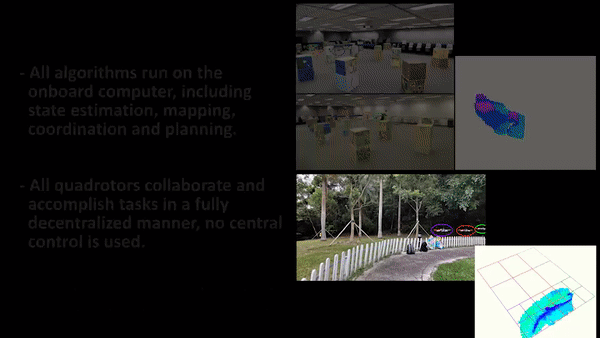
Our recent work toward fully automated and highly efficient aerial reconstruction, published at ICRA 2023, by Chen Feng. [Paper][Code][Video]
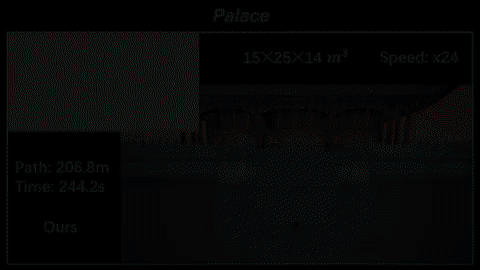
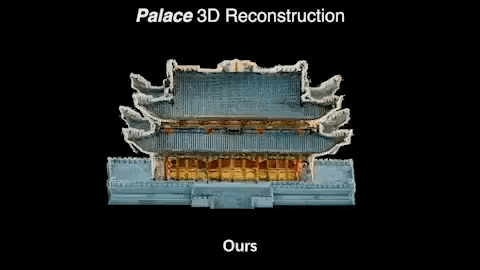